Agent-Based Models and Causal Inference in Computational and Quantitative Social Science

Agent-based models (ABMs) are a powerful tool for simulating complex social systems. They allow researchers to create virtual worlds in which they can experiment with different variables and observe the results. This can be a valuable way to gain insights into how social systems work in the real world.
However, ABMs can also be challenging to design and implement. One of the biggest challenges is causal inference. Causal inference is the process of determining the causal relationship between two or more variables. This can be difficult to do in ABMs because the models are often complex and nonlinear.
In this article, we will discuss the challenges of causal inference in ABMs and provide some tips for how to overcome them. We will also provide a step-by-step guide to designing and implementing ABMs for causal inference.
4.7 out of 5
Language | : | English |
File size | : | 769 KB |
Text-to-Speech | : | Enabled |
Screen Reader | : | Supported |
Enhanced typesetting | : | Enabled |
Print length | : | 174 pages |
Lending | : | Enabled |
There are a number of challenges to causal inference in ABMs. These challenges include:
- Complexity. ABMs are often complex and nonlinear. This can make it difficult to understand the relationships between the variables in the model.
- Uncertainty. ABMs are often based on uncertain data. This can make it difficult to determine the causal effects of the variables in the model.
- Bias. ABMs can be biased. This can be due to the way the model is designed or the data that is used to build the model.
There are a number of ways to overcome the challenges of causal inference in ABMs. These methods include:
- Design the model carefully. The design of the model is critical to causal inference. The model should be designed to be as simple as possible while still capturing the complexity of the system under study.
- Use high-quality data. The data used to build the model should be of high quality. This means that the data should be accurate, reliable, and unbiased.
- Validate the model. The model should be validated before it is used for causal inference. Validation involves comparing the model's predictions to real-world data.
- Use sensitivity analysis. Sensitivity analysis is a technique that can be used to assess the robustness of the model's results. Sensitivity analysis involves changing the values of the input variables in the model and observing the effects on the output variables.
- Use statistical methods. Statistical methods can be used to test the causal hypotheses that are generated by the model. These methods can be used to determine the significance of the causal effects and to estimate the size of the effects.
The following is a step-by-step guide to designing and implementing ABMs for causal inference:
- Define the research question. The first step is to define the research question that you want to answer with the ABM. The research question should be specific and focused.
- Develop a conceptual model. The next step is to develop a conceptual model of the system under study. The conceptual model should describe the key variables in the system and the relationships between them.
- Formalize the model. The conceptual model should be formalized into a computer model. The computer model can be written in a programming language such as Python or Java.
- Validate the model. The computer model should be validated before it is used for causal inference. Validation involves comparing the model's predictions to real-world data.
- Use the model to generate hypotheses. The computer model can be used to generate hypotheses about the causal relationships between the variables in the system under study.
- Test the hypotheses. The hypotheses can be tested using statistical methods. These methods can be used to determine the significance of the causal effects and to estimate the size of the effects.
ABMs are a powerful tool for simulating complex social systems. However, causal inference in ABMs can be challenging. By following the tips and steps outlined in this article, researchers can overcome the challenges of causal inference and use ABMs to gain valuable insights into how social systems work.
4.7 out of 5
Language | : | English |
File size | : | 769 KB |
Text-to-Speech | : | Enabled |
Screen Reader | : | Supported |
Enhanced typesetting | : | Enabled |
Print length | : | 174 pages |
Lending | : | Enabled |
Do you want to contribute by writing guest posts on this blog?
Please contact us and send us a resume of previous articles that you have written.
Novel
Text
Story
Genre
E-book
Newspaper
Paragraph
Shelf
Bibliography
Foreword
Scroll
Tome
Bestseller
Library card
Narrative
Biography
Autobiography
Memoir
Encyclopedia
Dictionary
Narrator
Character
Resolution
Borrowing
Archives
Periodicals
Study
Research
Scholarly
Lending
Academic
Special Collections
Interlibrary
Study Group
Thesis
Dissertation
Storytelling
Awards
Theory
Textbooks
Darrell Issa
Perla Bollo
Bob Sorge
Mounira M Charrad
Walter De La Mare
Diane Duane
Suzanne Falter
Steven C Wheelwright
Barbara Ebel
Fred Fanning
Rory Miller
Cindy Milstein
Benjamin Wittes
Buzz Walneck
Barbara Kaiser
Sebastian Harth
Lyn Cote
Lisa Yee
J Robin Maxson
Clive Forth
Light bulbAdvertise smarter! Our strategic ad space ensures maximum exposure. Reserve your spot today!
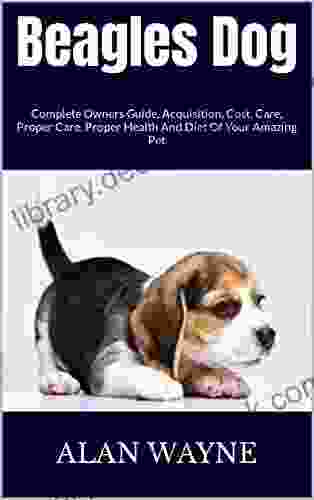

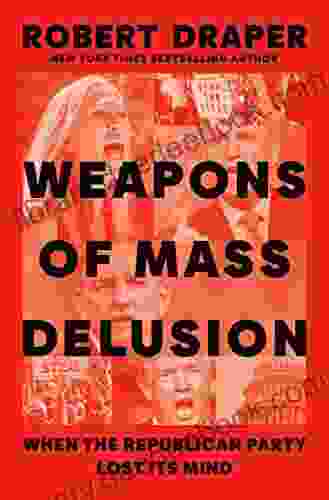

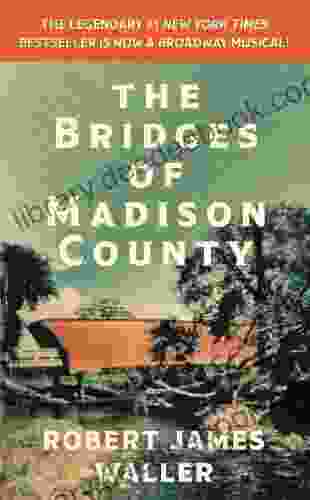

- Junichiro TanizakiFollow ·10.4k
- Elmer PowellFollow ·12.5k
- Octavio PazFollow ·14.9k
- Bernard PowellFollow ·11.4k
- Richard SimmonsFollow ·19.2k
- Fernando BellFollow ·14.2k
- Hudson HayesFollow ·5.7k
- Elias MitchellFollow ·9.3k
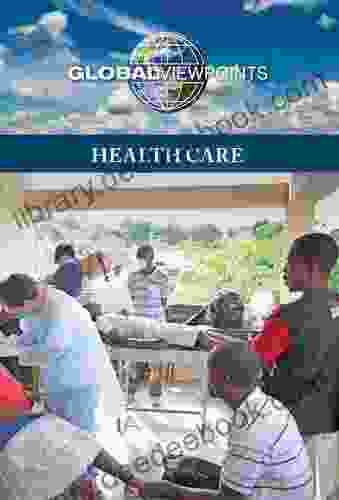

Health Care Global Viewpoints: Samantha Whiskey
Samantha Whiskey is a global health...
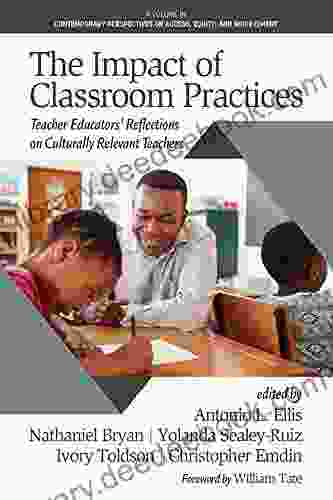

Teacher Educators' Reflections on Culturally Relevant...
In today's...
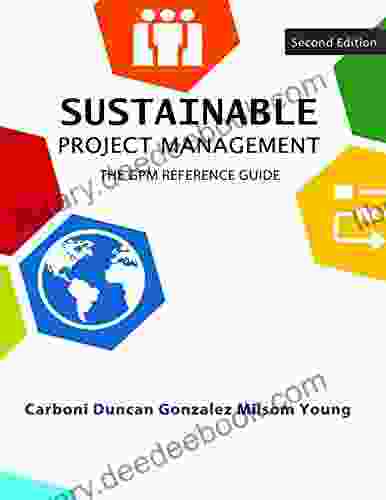

Sustainable Project Management: The GPM Reference Guide...
In today's rapidly changing world,...
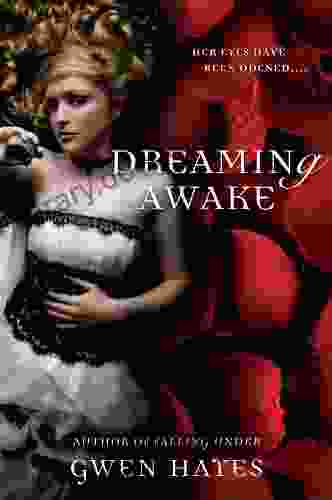

The Captivating World of "Dreaming Awake Falling Under"
A Journey Through...
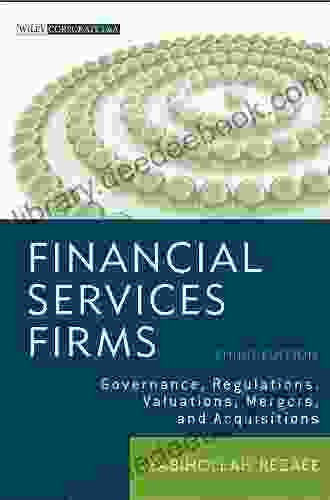

Governance Regulations Valuations Mergers And...
In today's complex and ever-changing...
4.7 out of 5
Language | : | English |
File size | : | 769 KB |
Text-to-Speech | : | Enabled |
Screen Reader | : | Supported |
Enhanced typesetting | : | Enabled |
Print length | : | 174 pages |
Lending | : | Enabled |